Motion analysis in videos – Exploring Video Data
Advanced concepts in video data analysis
The following concepts are fundamental in video data analysis and are commonly applied in real-world machine learning applications. Let’s see those concepts briefly here. Please note that the implementation of some of these concepts is beyond the scope of this book.
Motion analysis in videos
Concept: Motion analysis involves extracting and understanding information about the movement of objects in a video. This can include detecting and tracking moving objects, estimating their trajectories, and analyzing motion patterns.
Tools: OpenCV (for computer vision tasks) and optical flow algorithms (e.g., the Lucas-Kanade method).
Let’s see the overview of the code for motion analysis in video data.
Initialization: Open a video file and set up parameters for Lucas-Kanade optical flow:
import cv2
import numpy as np
# Read a video file
cap = cv2.VideoCapture(‘/<your_path>/CricketBowling.mp4’)
# Initialize Lucas-Kanade optical flow
lk_params = dict(winSize=(15, 15), maxLevel=2, \
criteria=(cv2.TERM_CRITERIA_EPS |
cv2.TERM_CRITERIA_COUNT, 10, 0.03))
Feature detection: Detect good feature points in the first frame using the Shi-Tomasi corner detection algorithm:
ret, frame1 = cap.read()
prvs = cv2.cvtColor(frame1, cv2.COLOR_BGR2GRAY)
prvs_points = cv2.goodFeaturesToTrack(prvs, maxCorners=100, \
qualityLevel=0.3, minDistance=7)
Motion analysis loop: Iterate through video frames, calculating optical flow and drawing motion vectors on each frame:
while True:
ret, frame2 = cap.read()
if not ret:
break
next_frame = cv2.cvtColor(frame2, cv2.COLOR_BGR2GRAY)
# Calculate optical flow
next_points, status, err = cv2.calcOpticalFlowPyrLK( \
prvs, next_frame, prvs_points, None, **lk_params)
Visualization: Display the original frame overlaid with motion vectors in real time:
# Draw motion vectors on the frame
mask = np.zeros_like(frame2)
for i, (new, old) in enumerate(zip(next_points, prvs_points)):
a, b = new.ravel().astype(int)
c, d = old.ravel().astype(int)
mask = cv2.line(mask, (a, b), (c, d), (0, 255, 0), 2)
frame2 = cv2.circle(frame2, (a, b), 5, (0, 0, 255), -1)
result = cv2.add(frame2, mask)
cv2.imshow(‘Motion Analysis’, result)
Exit condition: Break the loop upon pressing the Esc key:
# Break the loop on ‘Esc’ key
if cv2.waitKey(30) & 0xFF == 27:
break
Cleanup: Release the video capture object and close all OpenCV windows:
cap.release()
cv2.destroyAllWindows()
This code provides a simple yet effective demonstration of motion analysis using optical flow, visualizing the movement of feature points in a video.
We get the following output:
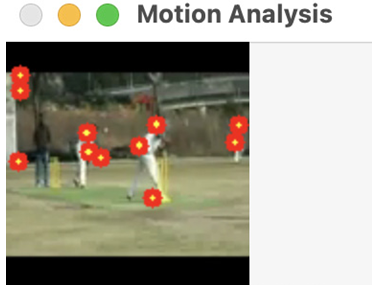
Figure 8.8 – Motion analysis in video
Leave a Reply